
Curiosity Lab
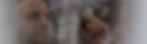
Ongoing Projects
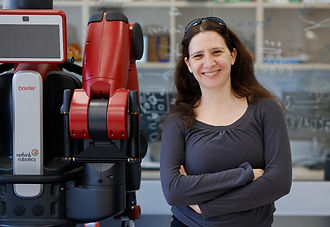
Project: Curiosity in data science – Curious Feature Selection (CFS)
By Michal Moran| ISF Funding
Intrinsic motivation refers to behavior driven by internal rewards. Intrinsically motivated computational learning, also known as artificial curiosity, causes an autonomous agent to act to optimize its learning about itself and its environment by receiving internal rewards based on prediction errors. This type of learning, which is based on the reinforcement learning paradigm, has been mostly implemented in developmental robotics, in which robots interact and learn about themselves and their environment to expedite learning compared to taking random actions.
Expedited learning in rapidly changing environments is also highly relevant in big-data science, where the process of building machine learning models can be very challenging due to continuous changes in data structures and the need for human interaction to tune the variables and models over time. This work presents a novel method of intrinsically motivated learning and curiosity loops to learn the data structures in large and varied datasets. An autonomous agent learns to select a subset of relevant features in the data (i.e., feature selection) to be used later for model construction. The agent optimizes its learning about the data structure over time without requiring external supervision.
Unlike other feature selection methods, which are restricted to either batch or online learning setting, the proposed method can be applied for both batch and online learning, it is not restricted to a specific predictor, and has other advantages such as running time and responsiveness to data changes. Experiments on three public datasets show that the proposed method, called the Curious Feature Selection (CFS) algorithm, positively impacts the accuracy of learning models using features selected by the CFS algorithm.
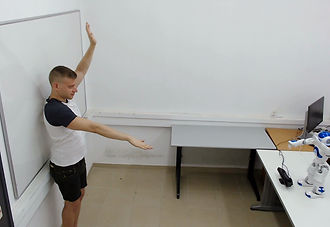
Project: Social Robots as Physical Curiosity Assessment Tools
By Matan epshtain | ISF Funding
Curiosity, the intrinsic drive to learn and the actions that satisfy that drive, is an maverick trait which is highly demanded today. however, the assessment of curiosity in adults is done today by self-reporting questionnaires which are biased by nature. By leveraging the unique characteristics of social robots we attempt to assess how people physically explore, by moving their arms, a social and cognitive learning task. In this study we implement a fully autonomous social robot which plays as the ``experimenter'', i.e. it gives instructions to the human subject on what to do next; to act as the learning task, i.e. people are asked to learn how to move the robot and; to be the assessor, i.e. it accumulates the objective measures of the subjects' exploration behavior. The complex interaction enables us to disambiguate learning, exploration and curiosity-based behaviors, where we show the highly significant correlation between our extracted behavioral measures and external self-reported personality traits and Psychometric Entrance Test (PET) scores.
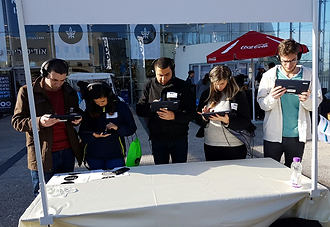
Project: Priming, Enabling and Assessment of Curiosity
By Keren Ben-Tov | ISF Funding
How easy is it to prime for curiosity and what are its effects? What simple measures can be used to enable for curiosity-driven behaviors? We have conducted a large-scale study to address these questions, using a novel curiosity-based app on university applicants. Using the same app, we addressed the issue of curiosity assessment, which in recent years has mainly been performed via self-reporting questionnaires. The curiosity assessment tool was developed in order to assess curiosity via an objective, quantitative and digital way. The tool measured several behavioral aspects during a free and task-less interaction with the tablet app. From the recorded activity logs we calculated quantitative behavioral measures related to their exploration patterns. We show that a single word can prime the participants and induce better learning of their self-explored knowledge. We also show that by simply enabling more time to explore, without the ability to stop at will, induces more exploration and more learning. Finally, we show that our behavioral measures, obtained with the digital quantitative assessment tool, accurately segment the participants' self-reported curiosity and Psychometric Entrance Test (PET) scores. These results suggest that simple priming for curiosity and enabling enough time to explore improve self-paced learning, and that a relatively simple and short interaction with a digital app can greatly improve state-of-the-art curiosity assessment.
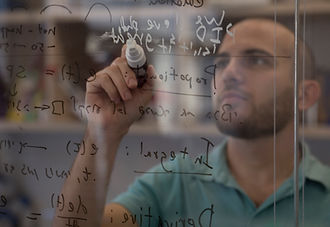
Project: quantum cognition
By Torr Polakow | BSF Funding
People are irrational beings, making decisions that defy classic laws of probabilities. On top of expressing multiple types of irrationalities, social interaction affects their instantiations in various
forms. In this project, we are modeling individual and social irrationalities in a unified formalism for a better understanding of human decision making, using quantum cognition.


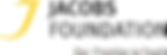
